Deep neural networks: researchers outsmart algorithms in order to understand them better
No one has yet managed to suss out just how artificial intelligence functions. Using subterfuge and all sorts of tricks, researchers are now trying to fathom the secrets of neural networks.
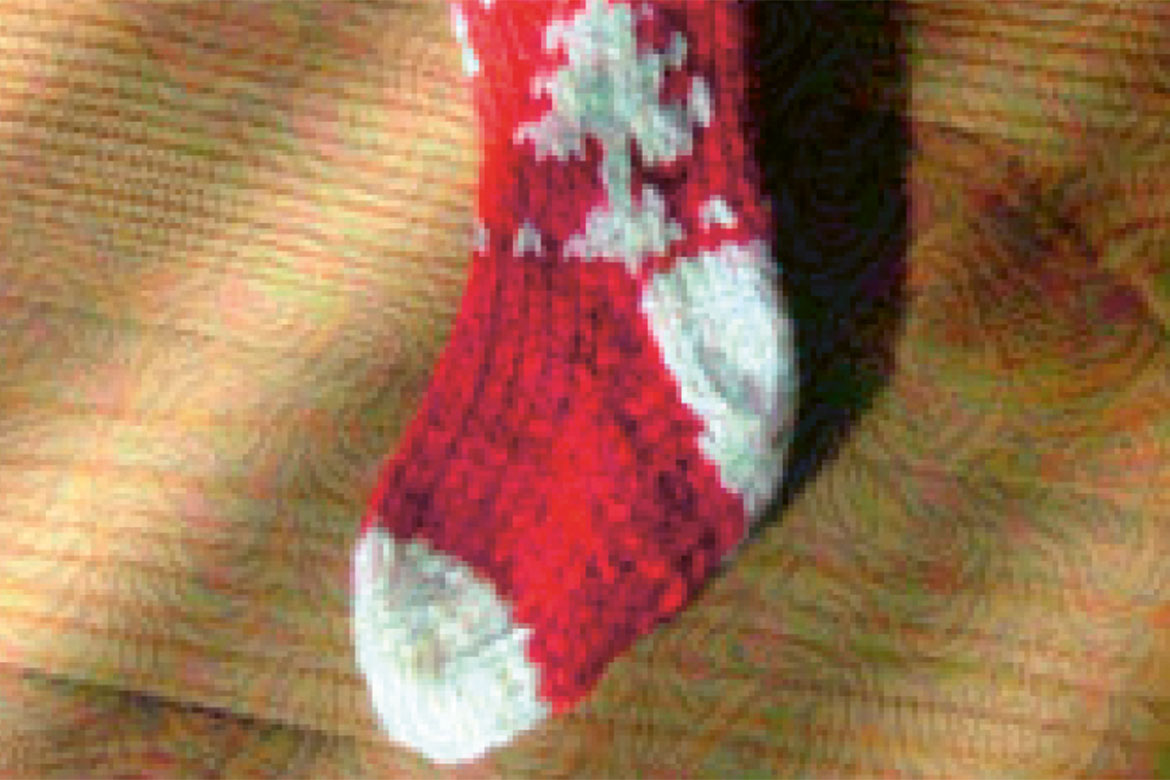
According to DNNs, this is the Indian elephant (Elephas maximus indicus).
Suddenly, experts have started to express unusual doubts about artificial intelligence. “Machine learning has become a kind of alchemy”, Ali Rahimi of Google recently prophesied in a lecture he gave. This provocation triggered a lively debate – he’d obviously hit a nerve.
Perhaps a setback was overdue. In recent years, we have been celebrating the astonishing successes of deep neural networks (DNNs), such as in speech and image recognition. DNNs are adaptive networks comprising several layers of virtual neurons. But now an unease has set in: Do we really know what’s happening inside neural networks? Can these new technologies be outsmarted? Are they a security risk? These topics are being investigated in brand-new research fields that call themselves ‘explainable artificial intelligence’ or ‘AI neuroscience’.
DNNs can in fact be deceived in many ways, as several researchers have now shown. Anh Nguyen at Auburn University, USA, for example, has constructed images that make not the slightest sense to people, but which DNNs unambiguously identify as depictions of certain animals.
Then there are so-called ‘adversarial’ tests that are even more duplicitous. Realistic-looking images are here subjected to minimal changes. The human eye can barely perceive the difference, but the DNN identifies these manipulated images as depicting a completely different object. A group led by Pascal Frossard at EPFL, for example, has succeeded in getting a DNN to recognise a sock as an elephant.
“Systems using DNNs are at present pretty vulnerable to alterations of basic data”, says Frossard. “Often, we can offer no guarantees for their performance”. This fact can lead to real problems in the fields of medicine and safety. Self-driving cars, for example, have to be able to recognise traffic signs reliably. They can’t let themselves be misled by manipulated data.
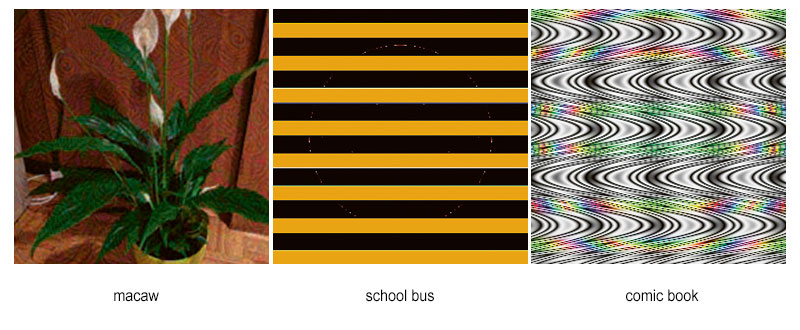
Confused neural networks: A sock becomes an elephant, a few lines are a school bus. Researchers have used these images to fool neural networks. | Images: S. Moosavi-Dezfooli, A. Fawzi, O. Fawzi and P. Frossard, Proceedings of IEEE CVPR, 2017 (Indian elephant, macaw); Nguyen et al., DNNs are Easily Fooled, CVPR 2015 (school bus, comic book)
Eight-legged zebras
Researchers are gradually beginning to understand why these mistakes occur. One reason is that the programs are trained with a limited volume of exemplary data. If they are then confronted with very different cases, things can sometimes go wrong. One further reason for their failure is the fact that DNNs don’t learn the structurally correct rendering of objects. “A real image of a four-legged zebra will be classified as a zebra”, explains Nguyen. “But if you add further legs to the zebra in the picture, the DNN tends to be even more certain that it’s a zebra – even if the animal has eight legs”.
The problem is that the DNNs ignore the overall structure of the images. Instead, their process of recognition is based on details of colour and form. At least this was the finding from the initial studies conducted into how DNNs function.
In order to suss out the secrets of neural networks, Nguyen and other researchers are using visualisation techniques. They mark which virtual neurons react to which characteristics of images. One of the results they found was that the first layers of DNNs in general learn the basic characteristics of the training data, as Nguyen explains. In the case of images, that could mean colours and lines, for example. The deeper you penetrate into a neural network, the more combinations occur among the information that has been captured. The second layer already registers contours and shadings, and the deeper layers are then concerned with recognising the actual objects.
There are astonishing parallels here with the neurosciences. Scientists have found indications that individual neurons in our brain could be specialised in reacting to specific people. Similar results have been found in DNNs.
Researchers are also trying to decode the inner life of neural networks by theoretical means. “For example, we are looking at the mathematical properties of algorithms”, explains Frossard. ‘Decision boundaries’ are the boundaries between different image categories. For example, a network will mark whether an image comes under the category of ‘apples’ or ‘pears’. But can such approaches, whether visual or theoretical, really serve to make the inner workings of DNNs sufficiently transparent?
As for their operating principles, many questions remain largely unanswered, says Yannic Kilcher from the Data Analytics Lab of ETH Zurich. This applies both to DNNs’ mistakes and to their miraculous successes. Often, even if a program is applied to unknown data, it will still provide reasonable results. “Why the neural networks should be capable of such generalisations is something we don’t yet fully understand”, says Kilcher.
Chess and tumours
In many applications, the sheer volume of data and the networked parameters make it very difficult to interpret the behaviour of DNNs. Even chess players struggle with the lack of transparency in programs that use DNNs. Recently, Google Alpha beat the best conventional chess computer program. But no one knows quite how it succeeded. If there are already problems in chess, what does it say about medical programs that help classify tumours? Are these so comprehensible and so tried-and-tested that we would be willing to rely on ‘decisions’ made by computer brains? Many researchers have doubts – even if they aren’t quite prepared to speak of ‘alchemy’.
The Defense Advanced Research Projects Agency (DARPA) of the US Department of Defense is already dealing with this challenge. In the project Explainable Artificial Intelligence, models are being developed that are based on DNNs, but are nevertheless transparent to the user. And researchers at Stanford University have developed a program that can investigate neural networks to find errors in them. It can also help us to better understand the decisions made by these DNNs. It succeeds when the complexity of the model is reduced to its essentials.
Frossard and his team are pursuing a different idea. They are introducing empirical prior knowledge to a DNN-supported model. The idea is that if you combine machine learning with concrete knowledge from the real world, then you might be able to construct a program that unites the best of both – the learning abilities of DNNs with the interpretability of conventional programs. Frossard says: “Ultimately, everything depends on the applications. But the best system is probably somewhere in the middle”.
The problem is that the reconstructed network can allow you to identify information about the confidential training data. If the data in question were patient data, for example, this would be especially awkward. Researchers such as Kilcher have been engaged in initial attempts to make such theft more difficult by means of skilful alterations to the programs.
Sven Titz is a freelance journalist and lives in Berlin.