Feature: AI, the new research partner
“Perhaps AI will one day write a better paper than I can”
Finding out how certain self-learning algorithms function is a painstaking task, says Claus Beisbart, a philosopher of science. He isn’t afraid of AI – in fact, he’s already using it as a tool in his own discipline.
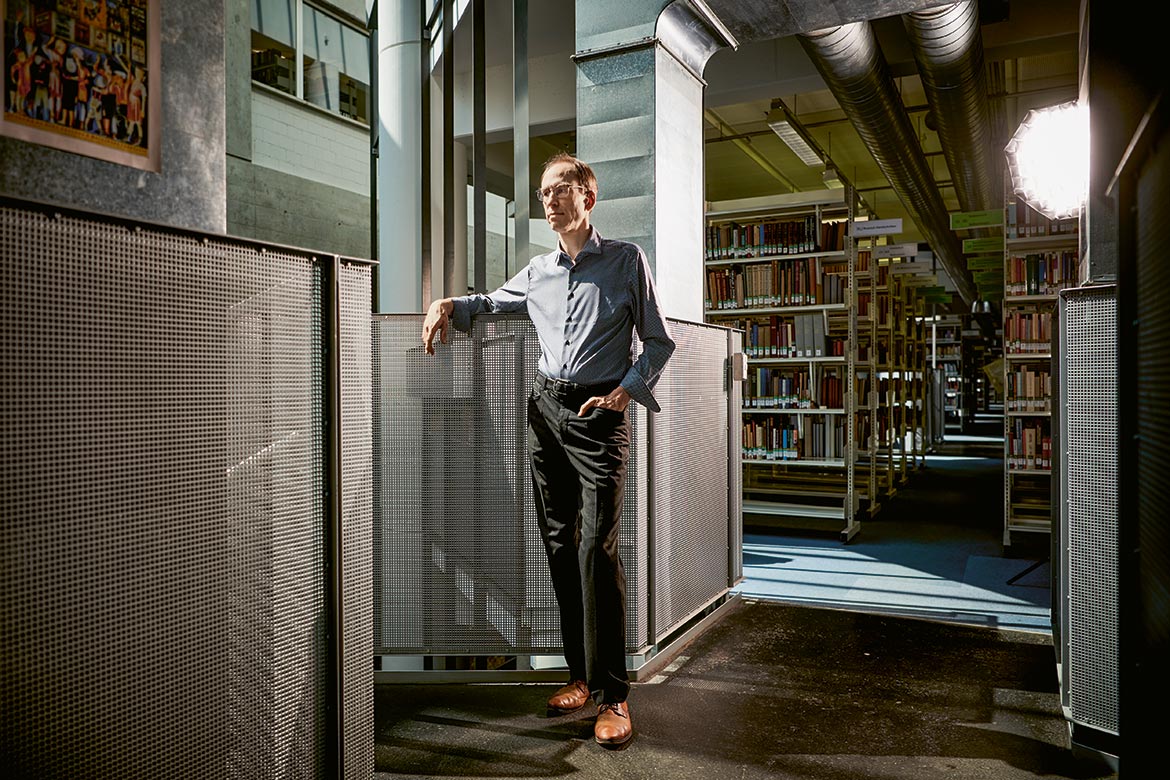
Claus Beisbart has no reservations about artificial intelligence. He even believes that humans and their computers together might become complex subjects of cognition. | Image: Raffael Waldner
Have you already tried to have a philosophical discussion with a chatbot?
Yes. We discussed how humans can preserve their autonomy and independence despite artificial intelligence – AI – becoming more and more powerful. ChatGPT used thoroughly relevant terms such as ‘human dignity’, ‘transparency’ and ‘fairness’. We’re still a long way off from having a deep philosophical conversation. ChatGPT barely stated any position of its own, for example. But all the same, we’re already using AI in philosophy.
Really? How can AI help you?
For example, we’re simulating whether a philosophical method can lead to consensus over contentious issues like eating meat. Of course, it all depends a lot on the structure of the debate. Either way, we don’t have any fear of working with the computer.
Can AI be described as a ‘black box’ that makes calculations so complicated that ultimately no one understands what’s actually happening inside?
That is undoubtedly a character trait of the new AIs, but it doesn’t describe them. There’s also the good, old-fashioned AI, which includes simulations such as are used by climate scientists. The rules according to which AI works, its equations, are provided by the researchers. So they know the variables that their computer is using for its calculations. It’s different with self-learning programs. These make up their own rules, based on the data they have. Their applications are particularly difficult to figure out, to reconstruct and to examine critically.
Can you give us a concrete example?
First you train a neural network. To do this, you give it data that have been classified correctly. When it then distinguishes between pictures of dogs and cats, we don’t know what features it’s responding to in the pictures. It’s possible that it is in fact paying most attention to the background. Researchers are getting ever better at understanding what these neural networks are reacting to, but it’s still often a matter of trial and error and is therefore an incredibly laborious process.
Can a neural network understand anything? Such as ChatGPT?
This could actually occur at some point. But ultimately, it’s just a language model that’s behind ChatGPT that is based on which words are most likely to follow each other. So the bot is simply parroting what has been said most often on the Internet. This could suffice to pass the Turing Test in which a human being has to decide whether they are talking to a computer or to another human being. But achieving verbal behaviour that appears acceptable to the outside world doesn’t necessarily involve any understanding. John Searle has devised a thought experiment for this. Someone who does not understand Chinese sits in a closed room, is given Chinese texts and Chinese questions about them. They have a manual with the rules for dealing with Chinese characters. This means they can formulate answers without actually understanding the language.
So what do we mean by ‘understand’? – in the case of people, of course.
Many people think of the ‘eureka’ moment of insight when something just ‘clicks’. But this feeling is individual and can be deceptive. This is why we don’t rely on it in philosophy. Instead, we try to link understanding to specific abilities. In language, this means that I can explain the meaning of a sentence; it means that I know how to combine expressions in new ways. When trying to understand events like the French Revolution, we have to be able to create networks of information, to recognise connections and draw our own conclusions.
How do you assess the capabilities of ChatGPT?
ChatGPT offers a kind of average of the texts you can find on the Internet. It doesn’t weigh up statements found there according to the degree of credibility of the sources. You could naturally incorporate such an ability in it. Unlike humans, computers need an incredibly vast amount of data. But if I show a doll’s pram once to a child, it can recognise one every time thereafter.
Can we rely on the outputs of an artificial intelligence?
If I use neural networks to classify galaxies, I can see that it’s worked in the past and that I can trust their track record. This is the only possible justification for using a neural network. I know more when I use good, old-fashioned climate simulations. Today, we understand the fundamental processes in the atmosphere so precisely that we can also calculate scenarios that haven’t occurred in the last 10,000 years.
In 2009, the laboratory automatons Adam and Eve caused a sensation when they created hypotheses of their own and conducted experiments using yeast cells. We don’t hear much about that anymore. Will it take a lot more before we can start replacing researchers with AI?
Adam and Eve demonstrated successfully what is actually possible. But the hypotheses made by Adam all follow the same simple pattern. There are already far more complex cases. Last year, an AI was fed with data from NASA on planetary movements so that it could use them to generate a law for gravitational force just like Newton did, 350 years ago. It worked well. All the same, they gave the AI a specific framework. Newton also had to invent differential calculus at the same time. To be sure, this is where things are trending right now – but there’s still going to be plenty of research work for us humans in the near future. AI also creates a lot of work for us because we don’t understand it.
So researchers don’t need to worry about becoming unemployed?
There’s no doubt that AI can deliver surprises. But perhaps we shouldn’t be playing humans and AI off against each other. The real question to ask is this: Who or what is actually the subject in research? Is it a human being? Some people believe that this hasn’t been the case for a long time now: it’s groups of people who understand things. Perhaps we are now seeing the emergence of a complex subject of cognition: a human being and their computer.
Will there ever be an AI philosopher?
As a philosopher, I fundamentally have to remain open to things. I can’t rule out the possibility that there’ll one day be an AI that writes better papers than I can. Computers are in any case very well suited to some things, like logic. But there are also philosophical methods that I think will be difficult for a computer to explore, such as analysing one’s own experience. What’s more, just writing a good paper isn’t what it’s all about. You also have to stay up-to-date with the topics that will be relevant in the future. In this respect, we humans probably have an advantage. Overall, I feel positive. Together with computers, we can achieve things in research that were previously impossible.